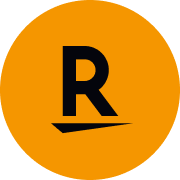
December 28, 2024
As organizations increasingly rely on data for decision-making, the complexity and scale of their data environments continue to grow. With this growth comes the challenge of ensuring data quality, integrity, and reliability across various systems, including data pipelines, cloud infrastructures, and hybrid environments. Traditional monitoring approaches can struggle to keep up with the dynamic nature of modern data systems, making the integration of Artificial Intelligence (AI) and Machine Learning (ML) in data observability a game-changer.
AI enhances data observability by enabling automated monitoring, anomaly detection, predictive analytics, and more. These technologies provide data teams with the tools needed to proactively manage data quality, improve performance, and resolve issues before they affect business outcomes. Here’s a closer look at how AI and ML are transforming data observability.
1. AI and Data Observability: A Perfect Match for Monitoring
Data observability is about gaining full visibility into your data infrastructure, including data pipelines, processing, and storage systems. While traditional monitoring tools provide basic insights, AI takes data observability to the next level by offering deeper, more actionable insights.
How AI Improves Observability:
- Predictive Analytics: AI algorithms can forecast potential issues before they occur, allowing data teams to take preventive actions.
- Automated Anomaly Detection: Machine learning models are trained to detect patterns in data, highlighting anomalies that may indicate data quality issues or pipeline failures.
- Root Cause Analysis: AI can analyze large datasets to quickly identify the root cause of issues, helping teams resolve problems faster.
By using AI to monitor and analyze data, organizations can achieve a level of insight and automation that was previously impossible with manual monitoring tools.
2. Data Observability vs. Monitoring: The Role of AI in Bridging the Gap
Data observability and traditional monitoring are often seen as similar concepts, but they serve distinct purposes. While monitoring focuses on tracking metrics and detecting failures, data observability goes beyond this by providing context and enabling teams to understand how data flows and transforms through their systems.
AI’s Contribution:
- Contextual Awareness: AI enhances monitoring by providing context around metrics, helping teams understand why issues are occurring and how to fix them.
- Proactive Monitoring: Instead of just alerting teams when something goes wrong, AI predicts potential failures and provides actionable insights to prevent them.
AI turns data observability into a proactive, intelligent system that continuously learns and adapts to new challenges, improving long-term outcomes.
3. AI and DataOps: Enhancing Collaboration and Speed
DataOps is a set of practices designed to improve collaboration and speed in data operations, similar to how DevOps improves software development. AI enhances DataOps by automating repetitive tasks, analyzing large datasets for insights, and optimizing data workflows in real-time.
Key AI Benefits for DataOps:
- Accelerated Decision-Making: AI tools analyze data in real-time, allowing teams to make faster, data-driven decisions.
- Automated Data Quality Checks: AI continuously monitors data for errors, inconsistencies, or gaps, ensuring high-quality datasets for analysis and modeling.
- Optimized Pipelines: By continuously learning from data, AI helps to optimize data pipelines, reducing manual intervention and improving efficiency.
By integrating AI with DataOps, organizations can streamline their data operations, improve collaboration between teams, and speed up the delivery of data insights.
4. AI for Anomaly Detection and Data Downtime Prevention
Data downtime and anomalies can have a significant impact on decision-making and business operations. AI helps prevent these issues by using advanced anomaly detection techniques to spot irregularities in data before they become serious problems.
Benefits of AI-Powered Anomaly Detection:
- Early Detection: AI models can detect anomalies in real-time, enabling teams to resolve issues before they affect data quality or business processes.
- Reduced False Positives: Machine learning models continuously improve, reducing the occurrence of false alarms and focusing attention on genuine issues.
- Faster Resolution: By automatically identifying the root cause of anomalies, AI accelerates the troubleshooting process, reducing downtime.
AI-powered anomaly detection is a critical component of data observability, allowing teams to act quickly and prevent disruptions.
5. AI in Cloud and Hybrid Cloud Environments
As more organizations move to cloud-based and hybrid data infrastructures, the complexity of managing and monitoring these environments grows. AI-driven observability tools are essential for managing the intricacies of cloud systems, providing a unified view of data health, performance, and security across multiple platforms.
How AI Supports Cloud and Hybrid Environments:
- Cross-Platform Visibility: AI integrates data from various cloud and on-prem systems, providing a comprehensive view of data health and performance.
- Scalable Monitoring: AI systems automatically scale with your infrastructure, adjusting to increasing data volumes without manual intervention.
- Optimized Resource Allocation: Machine learning algorithms predict resource needs, optimizing the allocation of cloud resources and preventing bottlenecks.
In cloud and hybrid environments, AI makes data observability more efficient, scalable, and adaptable.
6. Scaling Data Observability for Big Data Pipelines
Big data pipelines often handle large, complex datasets across distributed systems, making it challenging to ensure data quality and performance. AI can scale data observability to meet the demands of big data environments, providing real-time insights into pipeline health and data quality across multiple nodes and systems.
How AI Enhances Big Data Observability:
- Efficient Data Processing: AI optimizes big data processing by automating tasks such as data cleansing, validation, and transformation.
- Real-Time Pipeline Monitoring: AI continuously monitors big data pipelines, identifying and addressing issues in real time to prevent disruptions.
- Predictive Scaling: AI algorithms anticipate data spikes, scaling infrastructure resources in advance to ensure smooth operation.
With AI, organizations can scale their data observability strategies to handle the challenges of big data pipelines effectively.
7. The Future of Data Observability with AI
The integration of AI into data observability is not just a current trend—it’s shaping the future of how data systems are monitored and managed. As AI technology advances, the capabilities of data observability tools will continue to improve, offering even more advanced features like automated self-healing, deeper predictive analytics, and enhanced collaboration across teams.
Future Trends to Watch:
- Self-Healing Systems: AI will enable systems to automatically fix issues without human intervention, making data observability more autonomous.
- Deeper Integration with Data Governance: AI will enhance data governance by continuously monitoring data lineage, access, and compliance.
- Advanced Predictive Capabilities: AI will become even more accurate at predicting issues and bottlenecks, allowing teams to take preventive actions sooner.
As AI continues to evolve, it will play a central role in the future of data observability, enabling smarter, faster, and more efficient data management strategies.
AI is transforming data observability by enabling smarter, more efficient monitoring of data pipelines, cloud systems, and big data environments. From predictive analytics to anomaly detection and automated issue resolution, AI enhances the visibility, reliability, and quality of data across the organization. As data systems become more complex, AI-powered observability tools will be essential for ensuring data health and driving data-driven decision-making.
Ready to harness the power of AI for your data observability strategy? Learn more about Rakuten SixthSense Data Observability.