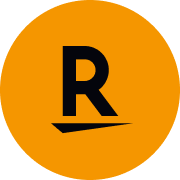
January 12, 2025
In today’s data-driven world, organizations need to ensure that their data flows seamlessly across systems, platforms, and teams. With vast amounts of data being generated every second, it’s essential to monitor and track key metrics to ensure data accuracy, reliability, and overall operational efficiency. This is where data observability tools come in.
Data observability tools provide the necessary visibility to understand and manage data pipelines, detect anomalies, and optimize performance. Whether you’re tracking system performance, data quality, or user interactions, knowing which metrics to monitor is crucial to ensuring your organization remains agile and efficient.
In this blog, we’ll discuss the top 10 metrics every organization should monitor using data observability tools. These metrics will help you stay on top of your data pipelines, ensure quality and integrity, and enhance decision-making across the board.
1. Data Pipeline Health
1.1 Understanding the Status of Data Pipelines
One of the core functions of data observability is to monitor the health of data pipelines. By continuously tracking the performance of your data pipelines, you can quickly detect disruptions or bottlenecks that could hinder data flow.
1.2 Key Indicators
- Pipeline Failure Rate: The percentage of failed data transfers or incomplete data processes.
- Latency: Time taken for data to move through each stage of the pipeline.
- Throughput: The volume of data processed per unit of time.
Monitoring these metrics will help organizations ensure their data flows smoothly, minimize downtime, and ensure seamless data operations.
2. Data Quality
2.1 Ensuring Accuracy and Consistency
Data quality is fundamental to making accurate business decisions. With data observability tools, organizations can track data integrity across their systems and ensure consistency and accuracy in real-time.
2.2 Key Indicators
- Data Completeness: Ensuring no data is missing or incomplete during transfers.
- Data Consistency: Ensuring the data is consistent across systems and platforms.
- Data Accuracy: Verifying that the data is accurate and free of errors.
By continuously monitoring these metrics, businesses can ensure high-quality data and prevent errors that may negatively affect operations or analytics.
3. Anomaly Detection
3.1 Identifying Irregularities in Data
Anomaly detection is one of the most powerful features of data observability tools. By automatically identifying unusual patterns in data, organizations can detect problems early, whether it’s a sudden drop in traffic, an unexpected change in user behavior, or an error in the data pipeline.
3.2 Key Indicators
- Anomaly Score: A numeric representation of how unusual a specific data point is based on historical data.
- Trend Deviation: The variance of data trends from expected patterns.
- Outlier Detection: Identifying data points that deviate significantly from the mean.
Real-time anomaly detection helps organizations resolve issues before they escalate, ensuring data-driven decisions remain accurate and reliable.
4. System Performance Metrics
4.1 Monitoring Application and Database Performance
To ensure smooth data operations, monitoring system performance is crucial. Key metrics such as server response times, database query performance, and uptime are critical for identifying bottlenecks or failure points that can disrupt data flow.
4.2 Key Indicators
- Response Time: The time taken for systems or databases to respond to queries.
- Server Uptime: The total time the system or service is fully operational.
- Resource Utilization: Tracking how much CPU, memory, and bandwidth are being used.
These metrics provide insight into the health of the systems supporting your data infrastructure.
5. Data Latency
5.1 Tracking the Delay Between Data Generation and Use
Data latency refers to the delay between data generation and its use or transfer across systems. Latency issues can lead to outdated or delayed insights, affecting business decisions. By tracking latency, organizations can identify bottlenecks and optimize data flow.
5.2 Key Indicators
- End-to-End Latency: The total time it takes for data to move from its source to its destination.
- Network Latency: Delay caused by the network infrastructure.
- Processing Latency: Delay caused during data transformation or enrichment.
By identifying and addressing latency, businesses can improve the real-time processing of data and make timely decisions.
6. Data Consistency Across Systems
6.1 Ensuring Uniform Data Across Platforms
Data consistency is critical for maintaining reliable insights across various tools and platforms. Monitoring data consistency ensures that there are no discrepancies or conflicts between multiple data sources.
6.2 Key Indicators
- Data Synchronization: The process of ensuring data is updated uniformly across systems.
- Data Integrity: Ensuring that the data remains intact and uncorrupted as it moves through different systems.
- Consistency Score: A measure of the consistency between data in different systems.
Tracking data consistency helps prevent errors, especially in complex systems where data is flowing from multiple sources.
7. Data Completeness
7.1 Ensuring All Necessary Data is Available
Data completeness refers to whether the full dataset is available for analysis or operational purposes. Missing or incomplete data can lead to flawed analyses, so monitoring this metric is critical.
7.2 Key Indicators
- Missing Data Alerts: Notifications triggered when expected data is not available.
- Data Availability: The percentage of required data successfully collected from sources.
- Null Value Rate: The frequency of missing or null values in the dataset.
By ensuring data completeness, organizations can avoid decisions based on partial or inaccurate data.
8. Data Access Control Metrics
8.1 Tracking Permissions and Access Levels
Data access control is essential for ensuring the security and integrity of organizational data. By monitoring access control metrics, organizations can ensure that sensitive data is only accessible by authorized personnel.
8.2 Key Indicators
- Access Request Volume: Number of requests made to access data.
- Unauthorized Access Attempts: Monitoring failed access attempts.
- User Roles and Permissions: Tracking who has access to what data.
This helps prevent data breaches and ensures compliance with data governance policies.
9. Data Usage Metrics
9.1 Understanding How Data is Used
Tracking data usage is essential for understanding how frequently and efficiently data is being accessed and used. This can help organizations optimize their data storage and ensure that valuable data is not underutilized.
9.2 Key Indicators
- Data Query Frequency: How often data is queried or used.
- Data Access Patterns: Tracking how different teams or departments use data.
- Data Retention: How long data is retained before being archived or deleted.
By understanding data usage, organizations can better allocate resources and improve the efficiency of their data-driven decision-making.
10. Data Governance Metrics
10.1 Ensuring Compliance and Risk Management
Effective data governance is essential for compliance with regulations and minimizing operational risks. Monitoring data governance metrics ensures that data is being used responsibly, securely, and in line with compliance standards.
10.2 Key Indicators
- Compliance Score: A measure of how well the organization adheres to data governance policies.
- Audit Trail: The tracking of changes made to data and its access.
- Data Lineage: Mapping the flow and transformation of data to ensure traceability and accountability.
Data governance metrics help ensure that data remains secure and compliant across the entire organization.
With the growing complexity of modern data ecosystems, it’s crucial for organizations to leverage data observability tools to monitor key metrics effectively. Tracking these metrics helps organizations identify issues early, optimize performance, and improve decision-making. By leveraging data observability, businesses can ensure they’re operating efficiently, securely, and with high-quality data.
Ready to enhance your organization’s data observability strategy? Try Rakuten SixthSense for a demo today!